The Eye as a Window to AI in Healthcare
The term Artificial Intelligence (AI) was first coined by John McCarthy in 1956 when he defined the concept as “the science and engineering of making intelligent machines.” (1)
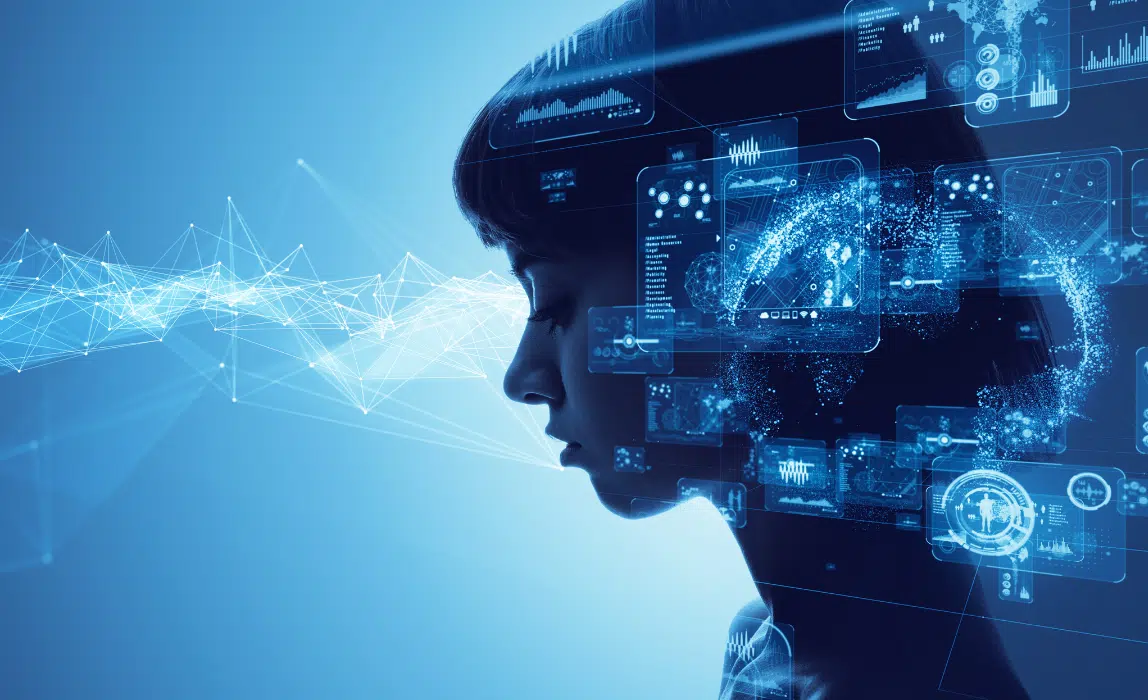
Since then, and especially in the last decade, important advances have led to various achievements including face recognition, natural language processing, speech recognition, text production, language translation, and more. (2) However, the most crucial AI developments have been made in healthcare with the goal to improve patient care by accelerating processes, increasing accuracy, and enhancing overall patient health. (3)
Biomarkers in the Eye
To make an impact in healthcare, AI and Machine Learning (ML) need to identify new ways for the practitioner to pose a diagnosis and establish unsuspected correlations. To do so, algorithms rely on the extraction and analysis of biomarker data. Biomarkers, or biological markers, are objective indications of a medical state (as opposed to symptoms reported by the patient) that can be measured and reproduced accurately. (4) Biomarkers provide a better understanding of the body and how it works, with applications notably in risk stratification, diagnosis, prognosis, and therapy monitoring.
The eye is an ideal location to identify, validate and leverage biomarkers due to its transparent properties enabling noninvasive access to different structures related to ocular, brain, and systemic health.
The Eye: A Window Into Health
Since the invention of the ophthalmoscope in 1851, physicians have been viewing the inside of the eye. (7) Through the pupil, a clinician can directly view, among other things, blood vessels and the optic nerve, a direct extension of the brain. To assess these key structures, fundus photography rapidly became standard practice in eye care and an important generator of health data. With the proper tags from the clinician, AI and ML aim to facilitate the diagnosis of common eye conditions and increase the breadth of clinical information we can obtain from conventional fundus imaging. For instance, AI-driven analysis of fundus images can spot early diabetic retinopathy in asymptomatic patients and has also been successful at detecting other ocular conditions such as age-related macular degeneration, glaucoma, and retinopathy of prematurity. In 2018, the FDA cleared IDx-DR, the first system that uses artificial intelligence (AI) to detect “greater than mild” diabetic retinopathy (DR) in adults with diabetes. In a study, IDx-DR correctly identified the presence of more than mild diabetic retinopathy 87.4% of the time and correctly identified those who did not have more than mild diabetic retinopathy 89.5% of the time. (4) Deep-learning models have also been able to analyze the data from the fundus photography of 284,335 patients and predict cardiovascular risk factors such as age, gender, smoking status, systolic blood pressure, and major adverse cardiac events. The models used anatomical features, such as the optic disc or blood vessels, to generate predictions. (13)
Having a direct link to the brain, the eye is often used as a non-invasive entrance for the study and analysis of neurological biomarkers. Several studies using the analysis of eye movements (9) highlight ML’s potential to predict behavior, cognitive states, and personality traits. (8) AI can draw conclusions about one’s personality traits (8) and even detect if they are lying by analyzing eye movements (12). Informative characteristics can be automatically determined from a large and diverse set of eye movement characteristics and patterns, uncovering previously unknown links between personality and gaze. Using machine learning and a set of features encoding different eye movement characteristics, software was able to reliably predict four of the Big Five personality traits (neuroticism, extraversion, agreeableness, conscientiousness) as well as perceptual curiosity. (8) Eye movements may also be used to monitor disease progression and therapy efficacy in brain disorders like autism, Alzheimer’s, schizophrenia, neglect, and multiple sclerosis. (10) For the latter disease, the FDA has most recently granted “Breakthrough Device” designation to Innodem Neurosciences for its device ETNA™ which is intended to record eye movements for use in estimating disease severity in Multiple Sclerosis patients. (21)
Data collection in the eye has often been limited to imaging. The use of diffuse reflectance spectroscopy may bring data collection in the eye to a whole new level.
The Use of Spectroscopy in Eye Care
Data collection in the eye has often been limited to imaging. The use of diffuse reflectance spectroscopy (DRS) may bring data collection in the eye to a whole new level. By leveraging the entire light spectrum, it is now possible to analyze the optical characteristics of biological tissues with unprecedented precision levels. (14) It is already widely used in other fields of medicine including microbiology and pharmaceutical analysis. (15) Spectral imaging expands the capabilities by combining spectroscopy and imaging, providing both spectral and structural information. (16)
One promising application of spectroscopy in the eye is ocular oximetry. Ocular oximetry uses spectroscopic analysis to investigate the hemodynamics of the eye. Specifically, it is a direct, non-invasive technique that assesses the oxygen content in the tissue of the retina. Hemoglobin, a protein found in red blood cells, transports the oxygen in blood to the tissues. Retinal oximetry analyzes the distinct spectral profiles of both oxygenated and deoxygenated hemoglobin to determine oxygen saturation. (17)
Study data tends to indicate that retinal oxygen saturation is altered in a wide variety of ocular and systemic diseases. For instance, abnormalities of the retinal blood supply have been implicated in primary open-angle glaucoma, diabetic retinopathy, age-related macular degeneration as well as artery/vein occlusion. (20)
Conclusion
Using the eye as a window, AI-driven biomarkers generate unthinkable wealth of information with true potential for changing the way we approach diagnostics and monitoring of multiple diseases. The convergence of big data, AI, and ocular biomarkers study is bound to provide the most complete, most advanced assessment of ocular, neurologic, and systemic health, enabling early detection and better management of various diseases.
References
(1) Mintz Y, Brodie R. Introduction to artificial intelligence in medicine. Minim Invasive Ther Allied Technol. 2019 Apr;28(2):73-81. doi: 10.1080/13645706.2019.1575882. Epub 2019 Feb 27. PMID: 30810430.
(2) SDS Club. Infographic: The 21st Century’s AI Biggest Achievements. https://sdsclub.com/21st-century-ai-biggest-achievements/. Published October 7, 2021. Accessed June 27, 2022
(3) Schmidt-Erfurth, U, Sadeghipour, A, Bianca, S et all. Artificial intelligence in retina. Progress in Retinal and Eye Research. Volume 67, 2018, Pages 1-29,ISSN 1350 9462,https://doi.org/10.1016/j.preteyeres.2018.07.004.
(4) Review of Optometry. Artificial Intelligence Takes on Diabetic Retinopathy. https://www.reviewofoptometry.com/article/artificial-intelligence-takes-on-diabetic-retinopathy Published April 12, 2018. Accessed June 10, 2022
(5) Strimbu K, Tavel JA. What are biomarkers? Curr Opin HIV AIDS. 2010 Nov;5(6):463-6. doi: 10.1097/COH.0b013e32833ed177. PMID: 20978388; PMCID: PMC3078627.
(6) Tamhane, M., Cabrera-Ghayouri, S., Abelian, G. et al. Review of biomarkers in ocular matrices: challenges and opportunities. Pharm Res 36, 40 (2019). https://doi.org/10.1007/s11095-019-2569-8
(7) Ivanišević M. First look into the eye. Eur J Ophthalmol. 2019 Nov;29(6):685-688. doi: 10.1177/1120672118804388. Epub 2018 Oct 7. PMID: 30295065.
(8) Hoppe S, Loetscher T, Morey SA, Bulling A. Eye movements during everyday behavior predict personality traits. Front Hum Neurosci. 2018 Apr 13;12:105. doi: 10.3389/fnhum.2018.00105. PMID: 29713270; PMCID: PMC5912102.
(9) Yamamori H, Yasuda Y, Ikeda M, Hashimoto R. Comparison of eye movements in schizophrenia and autism spectrum disorder. Neuropsychopharmacol Rep. 2020 Mar;40(1):92-95. doi: 10.1002/npr2.12085. Epub 2019 Nov 27. PMID: 31774635; PMCID: PMC7292215.
(10) Shaikh AG, Zee DS. Eye Movement Research in the twenty-first century-a window to the brain, mind, and more. Cerebellum. 2018 Jun;17(3):252-258. doi: 10.1007/s12311-017-0910-5. PMID: 29260439.
(11) Nam U, Lee K, Ko H, Lee JY, Lee EC. Analyzing facial and eye movements to screen for Alzheimer’s disease. Sensors (Basel). 2020 Sep 18;20(18):5349. doi: 10.3390/s20185349. PMID: 32961984; PMCID: PMC7570590.
(12) Gonzalez-Billandon J, Aroyo AM, Tonelli A, Pasquali D, Sciutti A, Gori M, Sandini G, Rea F. Can a robot catch you lying? A machine learning system to detect lies during interactions. Front Robot AI. 2019 Jul 31;6:64. doi: 10.3389/frobt.2019.00064. PMID: 33501079; PMCID: PMC7805987.
(13) Poplin R, Varadarajan AV, Blumer K, Liu Y, McConnell MV, Corrado GS, Peng L, Webster DR. Prediction of cardiovascular risk factors from retinal fundus photographs via deep learning. Nat Biomed Eng. 2018 Mar;2(3):158-164. doi: 10.1038/s41551-018-0195-0. Epub 2018 Feb 19. PMID: 31015713.
(14) Tomasz Kryczka, Edward Wylęgała, Dariusz Dobrowolski, Anna Midelfart, “NMR spectroscopy of human eye tissues: a new insight into ocular biochemistry”, The Scientific World Journal, vol. 2014, Article ID 546192, 9 pages, 2014.https://doi.org/10.1155/2014/546192
(15) Chakraborty, J, Das, S. Application of spectroscopic techniques for monitoring microbial diversity and bioremediation. Applied Spectroscopy Reviews. 08 Jun 2016, Published online: 26 Jul 2016. Doi: https://doi.org/10.1080/05704928.2016.1199028
(16) Mordant, D., Al-Abboud, I., Muyo, G. et al. Spectral imaging of the retina. Eye 25, 309–320 (2011). https://doi.org/10.1038/eye.2010.222
(17) Anupam K. Garg, Darren Knight, Leonardo Lando, Daniel L. Chao; Advances in retinal oximetry. Trans. Vis. Sci. Tech.2021;10(2):5. doi: https://doi.org/10.1167/tvst.10.2.5.
(18) DePaoli, D.T., Tossou, P., Parent, M. et al. Convolutional neural networks for spectroscopic analysis in retinal oximetry. Sci Rep 9, 11387 (2019). https://doi.org/10.1038/s41598-019-47621-7
(19) Shughoury A, Mathew S, Arciero J, Wurster P, Adjei S, Ciulla T, Siesky B, Harris A. Retinal oximetry in glaucoma: investigations and findings reviewed. Acta Ophthalmol. 2020 Sep;98(6):559-571. doi: 10.1111/aos.14397. Epub 2020 Apr 5. PMID: 32248646.
(20) Balyen, Lokman MD∗; Peto, Tunde MD, PhD† Promising artificial intelligence-machine learning-deep learning algorithms in ophthalmology, Asia-Pacific Journal of Ophthalmology: May-June 2019 – Volume 8 – Issue 3 – p 264-272 doi: 10.22608/APO.2018479
(21) Newswire. Etna device from the leading digital health & AI company Innodem Neurosciences receives FDA “Breakthrough” designation for multiple sclerosis. https://www.newswire.ca/news-releases/etna-tm-device-from-the-leading-digital-health-amp-ai-company-innodem-neurosciences-receives-fda-breakthrough-designation-for-multiple-sclerosis-811734861.html Published May 31, 2022. Accessed June 13, 2022
Written by the Zilia Team on June 30, 2022
More on our Blog
Zilia Partners with Kagawa University for Groundbreaking Retinal Oxygenation Study in Japan
Quebec City, Apr. 30, 2024 - Zilia, a pioneer in non-invasive ocular biomarker technologies, announces a...
Oxygen’s Complex Role in Retinopathy of Prematurity
Premature birth is a remarkable feat of medicine. However, this early entrance into the world can sometimes...
Oxidative Stress and Eye Health
Oxygen plays an important role in our universe, being the third most abundant element and the second most...
Solutions