How Artificial Intelligence is Transforming Ocular Health
Artificial intelligence (AI) applications are bound to revolutionize many industries. Eyecare is no exception, and this is why the Association for Research in Vision and Ophthalmology (ARVO) recently organized an online event to discuss how AI should alter and reshape the way diagnoses are made and treatments are provided.
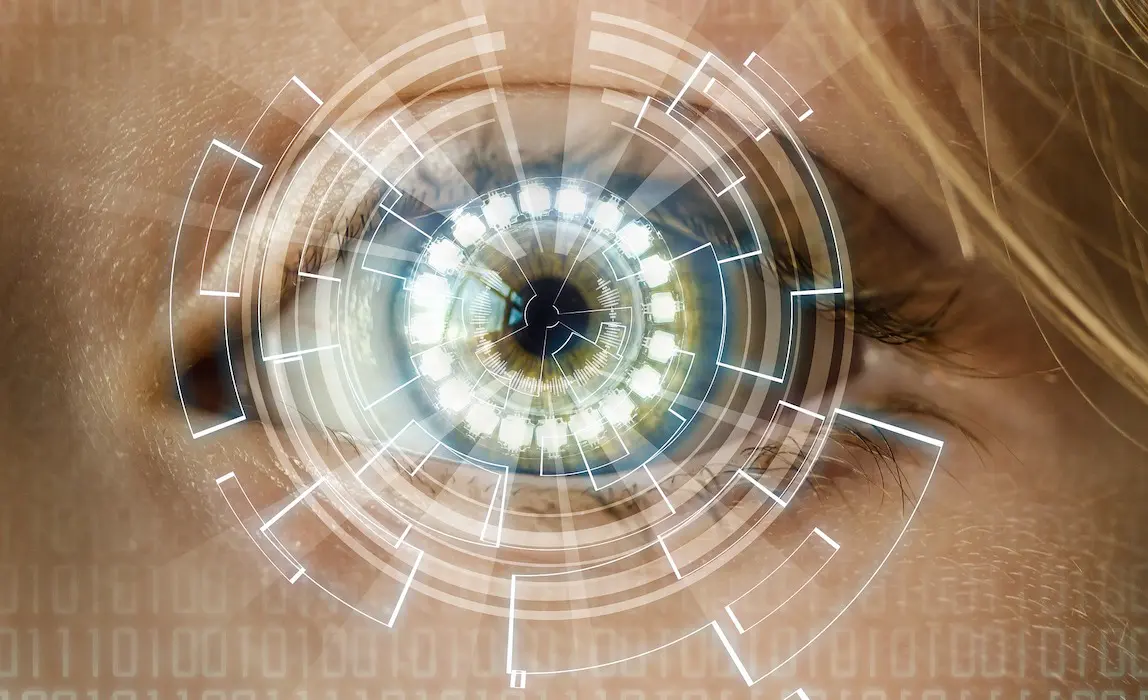
During the event’s Technology Showcase, Zilia had the opportunity to present how the combination of imaging, spectrometry, and its first AI-enabled device, called Zilia Ocular, can offer powerful insights about oxygen supply and ocular metabolism to eyecare professionals.
Improving ocular oximetry accuracy through AI
To this day, accuracy has been one of the most significant shortcomings in ocular oximetry (i.e., the assessment of oxygen saturation in the retina), limiting its clinical use for disease management. The traditional algorithmic technique used for calculating oxygen saturation from spectral information is linear regression. The light signal that returns from the eye fundus of the patient can be seen as a linear combination of the spectra of all its important photoabsorbent molecular constituents—e.g., oxygenated and deoxygenated hemoglobin. Linear regression thus aims to determine the individual contribution of each of these constituents. While it performs well in estimating oxygen saturation, it is susceptible to many factors, including noise, leading to reductions in precision and accuracy. Zilia uses a new algorithmic approach based on neural networks, which is significantly more robust, enabling higher levels of precision and accuracy.
“AI has the potential to be used for early diagnosis and management of ocular diseases such as glaucoma, diabetic retinopathy, and age-related macular degeneration.“
What is a convolutional neural network?
A convolutional neural network (CNN) uses a convolution operation to recognize specific patterns in, for example, a spectrum (i.e., a light signal). To do so, a filter (or kernel) moves iteratively across the spectrum in a convolutional manner and is compared to the spectrum. That way, the network learns to recognize a specific type of curve or trend, and returns a 1 if it is detected in the spectrum and a 0 otherwise. This combination of 0’s and 1’s is called an activation map (shown as the output in Figure 1) and indicates where the network has recognized a certain type of features. By convoluting different filters through the spectrum, we extract multiple types of features that are combined to obtain the oxygen saturation measurement.
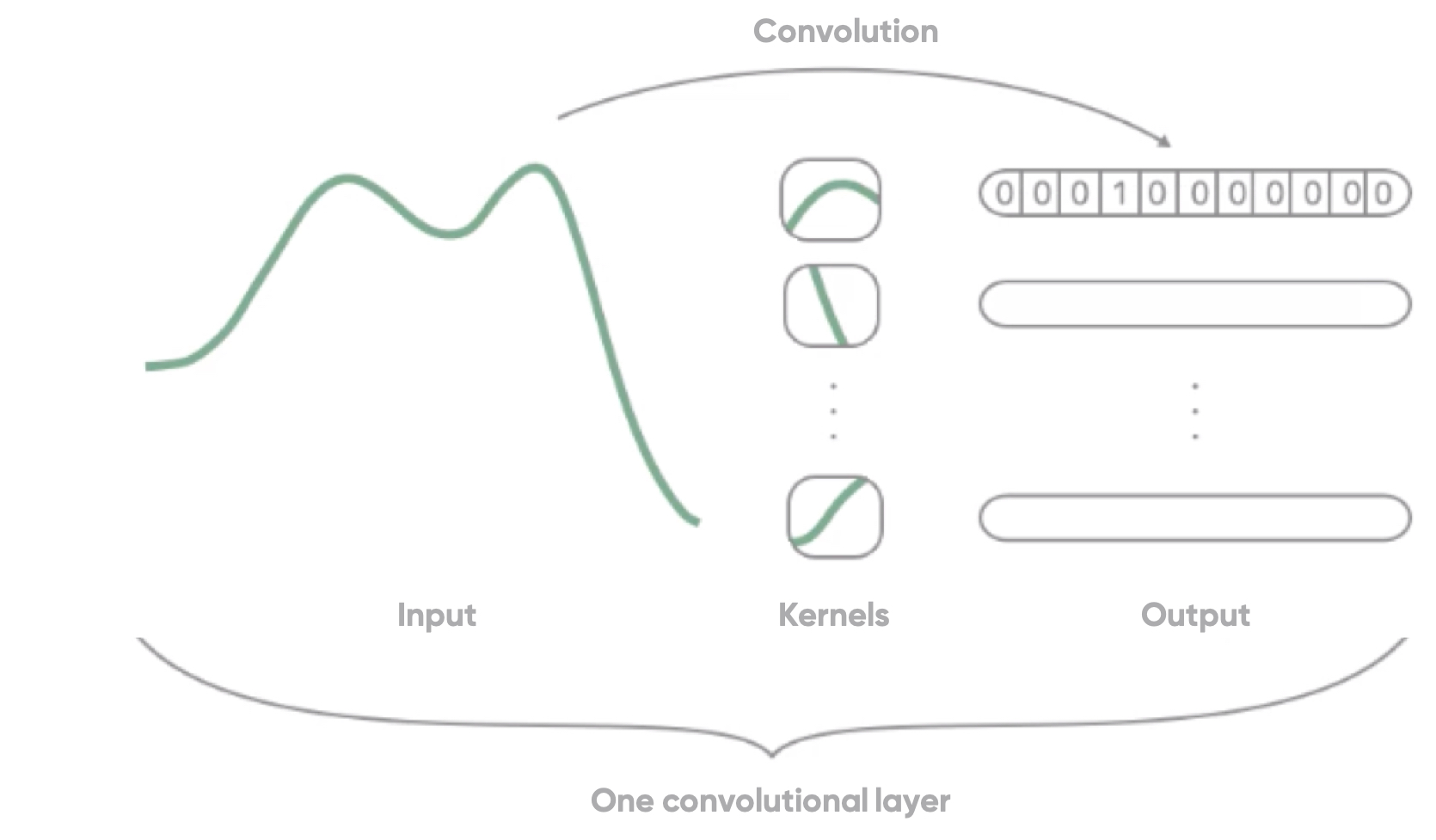
Figure 1: Schematic of a convolutional layer in a convolutional neural network
No ground truth
Since there is currently no means or technology to directly and ethically measure oxygen saturation in vivo in the tissue of the retina, there is no ground truth for ocular oximetry. On the other hand, to train a neural network, a lot of data is needed. Therefore, innovative ways were required to create datasets on which the network could be trained and tested.
Creating simulated data
Compounded spectra can be virtually created by combining the spectra of the different constituents present in the eye fundus (Figure 2 below) to simulate the range of spectra for different compositions in the blood. This way, the exact value of oxygen saturation becomes a known parameter, and this simulated data can be used to train CNN algorithms.
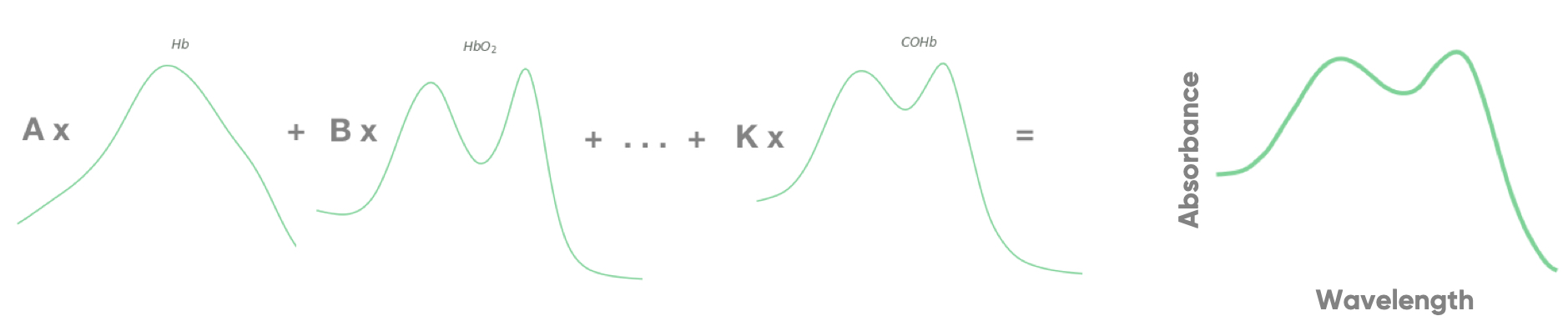
Figure 2: Simulated spectrum created from the combination of spectra of individual components present in blood.
A phantom eye, consisting of an in vitro system mimicking the optical properties of the human eye, with real oxygenated blood can then be used to test the resulting algorithm. The use of this phantom eye enables the measurement of blood oxygen saturation in the eye with Zilia Ocular and other reliable in vitro technologies as references. The resulting CNN trained on the simulated spectra and tested with the phantom eye performed better than traditional oximetry techniques, giving a lower mean error and standard deviation than algorithms based on linear regression.
AI to assess the autoregulation mechanism
Autoregulation is the adaptive mechanism by which the vascular system acts in response to different stimuli to regulate oxygen content in tissues. The variations in oxygen saturation over time in the retina (Figure 3) can give very valuable information related to the dynamics of autoregulation, which can be altered in many diseases. Combining this information from different features of the eye fundus, AI has the potential to be used for early diagnosis and management of ocular diseases such as glaucoma, diabetic retinopathy, and age-related macular degeneration. But it could go even further, potentially helping in the diagnosis and management of cardiological, neurological, and neurodegenerative diseases.
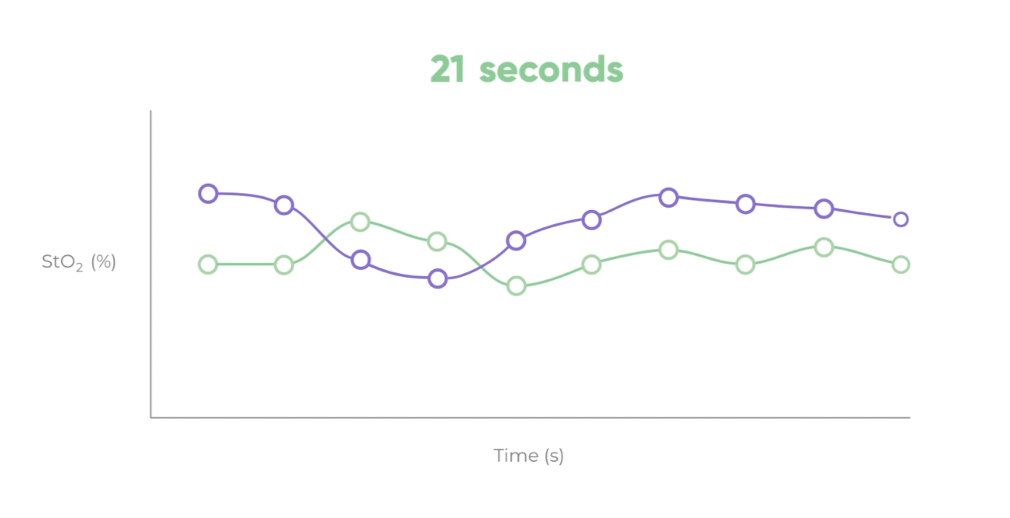
AI in computer vision
Zilia Ocular can acquire data in any feature of the eye fundus: the neuroretinal rim of the optic nerve head, the macula, blood vessels, and the retina. AI can be used to automatically detect these features using segmentation techniques, and therefore to determine oxygen saturation measurement very accurately in the eye fundus. Moreover, since structural changes are known to happen with disease progression, AI can provide an additional level of analysis for diagnosis and patient management. For instance, blood vessel segmentation in the eye and its characterization can be significantly improved by using CNNs compared to traditional techniques. Since ocular blood vessels can give important structural and functional information about vascular health, the performance of the segmentation is crucial.
Future applications for diagnosis and monitoring
The analysis of fundus images is the basis for many classical diagnostic techniques, and the implementation of AI in this field can greatly improve the analytical power. For example, retinal segmentation and characterization can be used to develop automated reports calculating general ocular health metrics and evaluating anatomical changes over time. All this can be combined with metabolic data to provide the most complete, most advanced assessment of ocular health, enabling early detection and better management of ocular diseases.
by Joannie Desroches, PhD on August 24, 2019
Further Reading
Zilia Launches Innovative Project to Detect a Rare Heart Disease
Quebec City, 15 May 2024 - Zilia, a Quebec-based medical technology company, announces the launch of a pilot...
Zilia Partners with Kagawa University for Groundbreaking Retinal Oxygenation Study in Japan
Quebec City, Apr. 30, 2024 - Zilia, a pioneer in non-invasive ocular biomarker technologies, announces a...
Oxygen’s Complex Role in Retinopathy of Prematurity
Premature birth is a remarkable feat of medicine. However, this early entrance into the world can sometimes...
Solutions